StAtIsTiCaL sIgNiFiCaNcE
How to handle the question of statistical significance in qualitative research
Of all the frustrating, irritating, and sometimes obnoxious questions hurled at folks doing qualitative research, the one that I dread the most is the infamous:
Are those findings statistically significant?
Even as I’m writing this I feel myself sneering at my screen. My blood is boiling, I’m fired up, and I’m going to yell at the wall.
The Statistical Significance Question is a Red Herring
In the past, having this question thrown my way has left me deflated. Not because it’s hard to answer - the literal answer is simply, “No”, with a side of “You’re missing the point.” It leaves me deflated because the person asking doesn’t understand what qualitative research is.
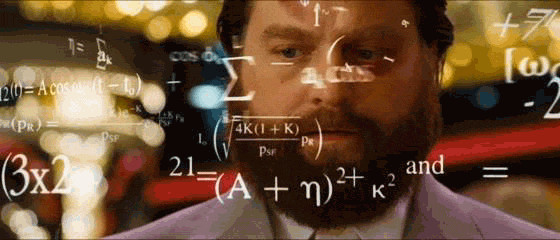
Qualitative research helps us understand how to think about things. Qualitative research through observation, interviews, or some other method provides us the opportunity to build new mental models that better represent what’s going on in the world. It drives new understanding of our world. Once those mental models have been established, we use quantitative research to evaluate if our models are reflective of reality, and then to build a more nuanced understanding of those models over time.
But you already knew that didn’t you? The problem isn’t your understanding of qualitative research, it’s the people you work with. How can we help them understand what statistical significance is and isn’t, and when it’s an appropriate tool to use?
Here’s an analogy I recently used (somewhat) successfully to explain, that combines my two favourite things: research, and basketball 🏀. After that, we’ll cover what you need to do differently next time you’re asked about statistical significance.
A Basketball Analogy
Let's say the defending NBA champion Toronto Raptors (🦖) sign free agent Lebron James in the offseason. After 5 games of the next season, we notice that the points scored per game are down compared to last season.
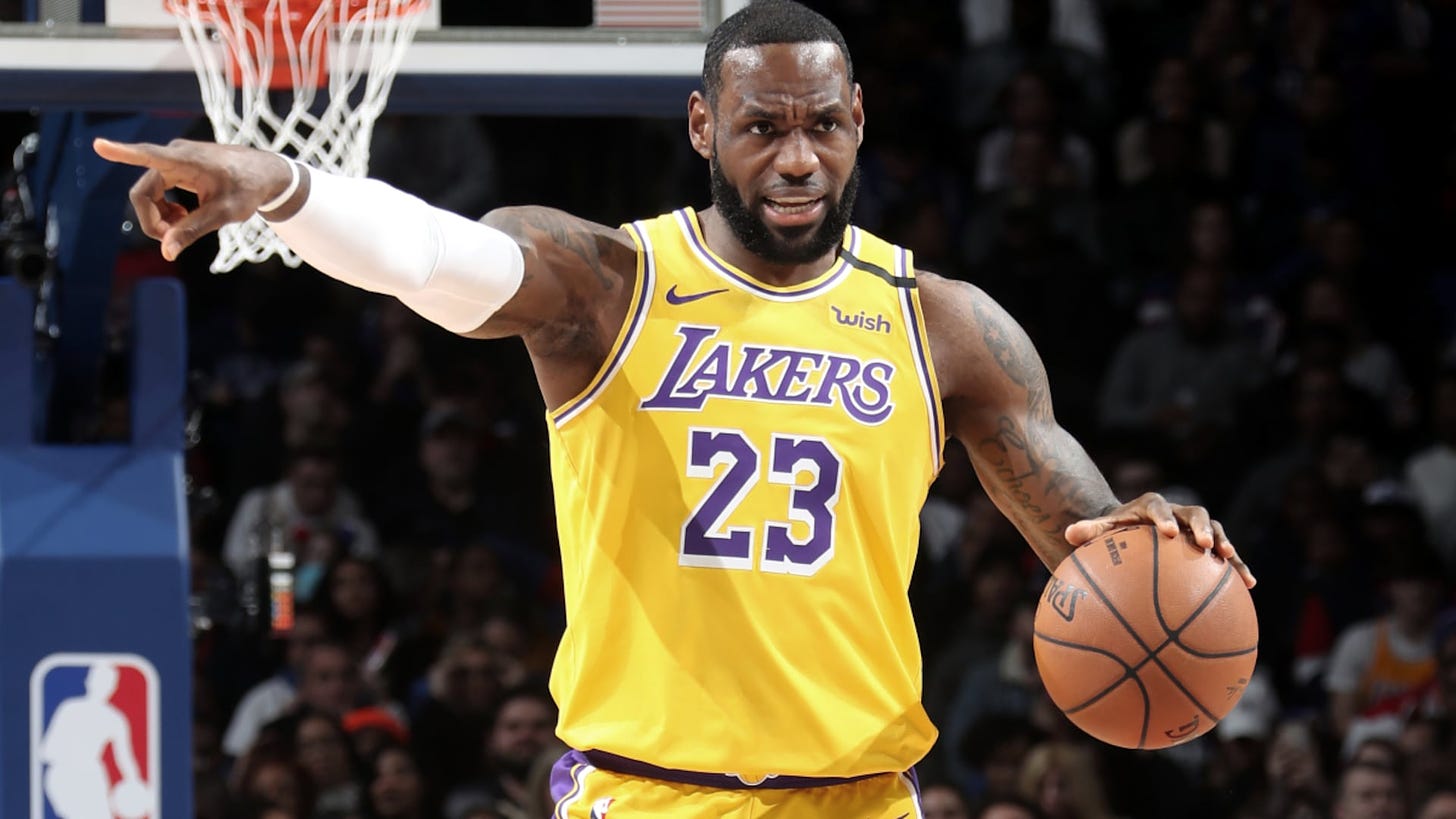
Now, we understand basketball pretty well, and we've seen players switch teams before. In fact, we've even seen Lebron James switch teams before, so we have a really good model of the factors at play here. Since we understand basketball well, and what happens when we integrate new players into teams, it makes sense to ask a quant question and consider the statistical significance of our measurements.
Now let's take that same situation, but instead of the Raptors signing Lebron James, its the Toronto Maple Leafs (🏒). 5 games into the season, things aren't going well and goals scored per game are down compared to last season.
Now we've never seen a player go from the NBA to the NHL, we don't know how familiar Lebron is with hockey, if he knows how to handle the puck, or how to forecheck. We do not have a model for how to think about this situation; it’s too different from anything we’ve seen before.
In this situation, before we can even consider the usefulness of measuring the statistical significance of wins and losses, we have to start by using qualitative methods to build a working mental model of the situation we’re in. We’d probably want to:
Interview LeBron about his relationship with hockey - how long has he been playing, why did he move to the NHL, what are his goals?
Interview and observe the team and management - have they ever integrated a player from another sport onto a hockey team, and how are they working together to improve?
Observe the team at practice - are line changes smooth, where is LeBron adding the most value, and are strong working relationships being developed between players on the team.
The knowledge gathered from those qualitative research projects will help us understand what to measure, how to measure it, and when it’s appropriate to measure statistical significance.
Your Job is to Flip the Question
Too often as researchers we answer questions literally rather than thinking about the intention behind the question.
When we share a meal with friends, and someone asks, “Does anyone else want that last slice of cake?”, we know that while they are literally asking if anyone else has the desire to consume more dessert, they are really are asking if it’s socially acceptable if they eat the last slice.
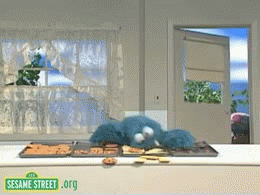
Let’s play the same game here, what is the real intention behind the question of statistical significance?
In my experience, most people don’t know the definition of statistical significance, or that the ‘p-value’ is somewhat arbitrarily chosen (usually at 5% of lower). Let’s head over to wikipedia for this one:
a result has statistical significance when it is very unlikely to have occurred given the null hypothesis
Null hypothesis is a fancy term that means there’s no difference between two or more groups you’re comparing. This is probably something not understood by your coworkers, so here’s what they are really asking:
Are your conclusions valid, can I trust this information, and does this apply to me?
Given that, how do we proceed? Well, there are two things we need to do to effectively disarm and realign our stakeholders with our research:
Rather than respond directly to questions of statistical significance, ask them about what their priorities and concerns are. Use this as a chance to build rapport and learn about your colleague’s aspirations and anxieties. Then, contextualize your research using their POV explaining its limitations and its appropriate application.
Bring coworkers into the research process by inviting them to help build a quantitive study that is helpful and builds on your qualitative work. Let’s face it, whether the data is quality or not there is comfort in large numbers. Design a study to measure statistical significance later on, and ask them to help you ideate on ways to do that.
One Last Thing…
I hope that analogy and advice is helpful to you in supporting the people you work with. However, this common conundrum is also an opportunity to look inward and be better.
While we spend so much time trying to build a better understanding of our customers, digging deep into the meaning behind their words rather than taking what they say at face value, we can’t forget to do this with our teams as well.
Put yourself in your colleagues’ shoes. It is perfectly reasonable to be anxious about a study using methods you are unfamiliar with influencing what you build. Recognize that as researchers we have power to exalt and the power to extinguish projects, promotions, and even people’s jobs.
Thanks for reading, until next time 🚀
alec
P.S. if you found this helpful, or have any feedback or suggestions for future posts please DM me on Twitter or email me at alec [at] uxrcollective [dot] com.